Welcome to MLatom documentation!
MLatom is a program package for AI-enhanced computational chemistry. It is designed to leverage the power of ML to speed up and make more accurate common simulations and to create complex workflows.
The users can run simulations with input files, Python script, and command-line options.
We provide:
MLatom – an open-source package that can be installed via
pip
and cloned from GitHub.A-MLatom – an advanced version with many state-of-the-art methods such as UAIQM which are often unpublished and not available in the open-source MLatom. You can purchase this version for local and fully private use or choose MLatom@XACS.
MLatom@XACS – a variant of A-MLatom which you can run online at the XACS cloud computing service (generous amount of computing time is free for academic use and you can purchase more computing time for heavy simulations).
Online courses Modern computational chemistry and AI with MLatom@XACS by Pavlo O. Dral.
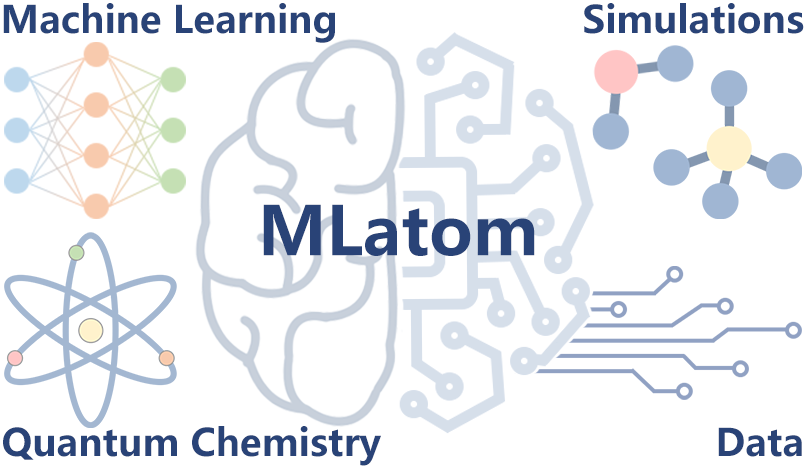
A video overview of the MLatom capabilities:
To get quickly started, please check a simple example illustrating the use of MLatom.
See a detailed overview of capabilities of MLatom for more information.
Tutorials: Introduction
Tutorials: Methods
Tutorials: (ML) models
Tutorials: Simulations
Tutorials: Excited states
Manual for input file/CLI
- Overview
- Simulations
- Single-point calculations
- Geometry optimization
- Frequencies and thermochemistry
- IRC
- Molecular dynamics
- IR and power spectra from MD
- Simulations with universal ML-based models
- Simulations with QM methods
- Simulations with user-trained models
- Quantum dynamics with machine learning
- UV/vis spectra
- Two-photon absorption cross sections
- Learning
- Data
Manual for API