面跳跃动力学
In this tutorial we show how to perform nonadiabatic molecular dynamics (NAMD) and analysis of NAMD trajectories with MLatom. The simulations are only possible through the Python API. MLatom currently only supports NAC-free Landau–Zener–Belyev–Lebedev (LZBL) surface hopping.
Running NAMD dynamics
See our paper for more details (please also cite it if you use the corresponding features):
Lina Zhang, Sebastian Pios, Mikołaj Martyka, Fuchun Ge, Yi-Fan Hou, Yuxinxin Chen, Joanna Jankowska, Lipeng Chen, Mario Barbatti, Pavlo O. Dral. MLatom software ecosystem for surface hopping dynamics in Python with quantum mechanical and machine learning methods. J. Chem. Theory Comput. 2024, 20, 5043–5057. DOI: 10.1021/acs.jctc.4c00468. Preprint on arXiv: https://arxiv.org/abs/2404.06189.
备注
Please refer to the tutorials of how to use ML for NAMD with ML models and perform active learning. The tutorial shown here will need some installations required to perform AIQM1 calculations, while NAMD with ML models will need minimum installations and can be performed online.
Here we show how to use AIQM1/MRCI in propagating LZBL surface-hopping dynamics. Please download the tutorial files (namd_aiqm1.zip
).
以下脚本展示了所有步骤:
优化几何构型
运行频率计算
使用Newton-X的例程从Wigner分布中生成初始条件。该示例可以扩展以支持按激发能量窗口进行筛选(有关更多详情,请参阅 手册 )。
并行传播多条轨迹(此处为16条轨迹,每条轨迹为5 fs,时间步长为0.1 fs)。
将轨迹储存为h5md格式
通过生成群体图来分析结果。
脚本如下:
import mlatom as ml
# Load the initial geometry of a molecule
mol = ml.data.molecule()
mol.charge=1
mol.read_from_xyz_file('cnh4+.xyz')
# Define methods
# .. for NAMD
aiqm1 = ml.models.methods(method='AIQM1',
qm_program_kwargs={'save_files_in_current_directory': True,
'read_keywords_from_file':'../materials/mndokw'})
# .. for optimization, frequencies and normal mode calculations
method_optfreq = ml.models.methods(method='B3LYP/Def2SVP', program='pyscf')
# Optimize geometry
geomopt = ml.simulations.optimize_geometry(model=method_optfreq,
initial_molecule=mol)
eqmol = geomopt.optimized_molecule
eqmol.write_file_with_xyz_coordinates('eq.xyz')
# Get frequencies
ml.simulations.freq(model=method_optfreq,
molecule=eqmol)
eqmol.dump(filename='eqmol.json', format='json')
# Get initial conditions
init_cond_db = ml.generate_initial_conditions(molecule=eqmol,
generation_method='wigner',
number_of_initial_conditions=16,
initial_temperature=0,
random_seed=1) # To ensure we always get the same initial conditions (should not be used in actual calculations)
init_cond_db.dump('test.json','json')
# Propagate multiple LZBL surface-hopping trajectories in parallel
# .. setup dynamics calculations
namd_kwargs = {
'model': aiqm1,
'time_step': 0.25,
'maximum_propagation_time': 5,
'hopping_algorithm': 'LZBL',
'nstates': 3,
'initial_state': 2, # Numbering of states starts from 0!
'random_seed': 1 # To ensure we always get the same initial conditions (should not be used in actual calculations)
}
# .. run trajectories in parallel
dyns = ml.simulations.run_in_parallel(molecular_database=init_cond_db,
task=ml.namd.surface_hopping_md,
task_kwargs=namd_kwargs,
create_and_keep_temp_directories=True)
trajs = [d.molecular_trajectory for d in dyns]
# Dump the trajectories
itraj=0
for traj in trajs:
itraj+=1
traj.dump(filename=f"traj{itraj}.h5",format='h5md')
# Analyze the result of trajectories and make the population plot
ml.namd.analyze_trajs(trajectories=trajs, maximum_propagation_time=5)
ml.namd.plot_population(trajectories=trajs, time_step=0.25,
max_propagation_time=5, nstates=3, filename=f'pop.png', pop_filename='pop.txt')
这是最终的群体图(由于初始条件和跃迁中的随机种子不同,您的图可能会有所不同):
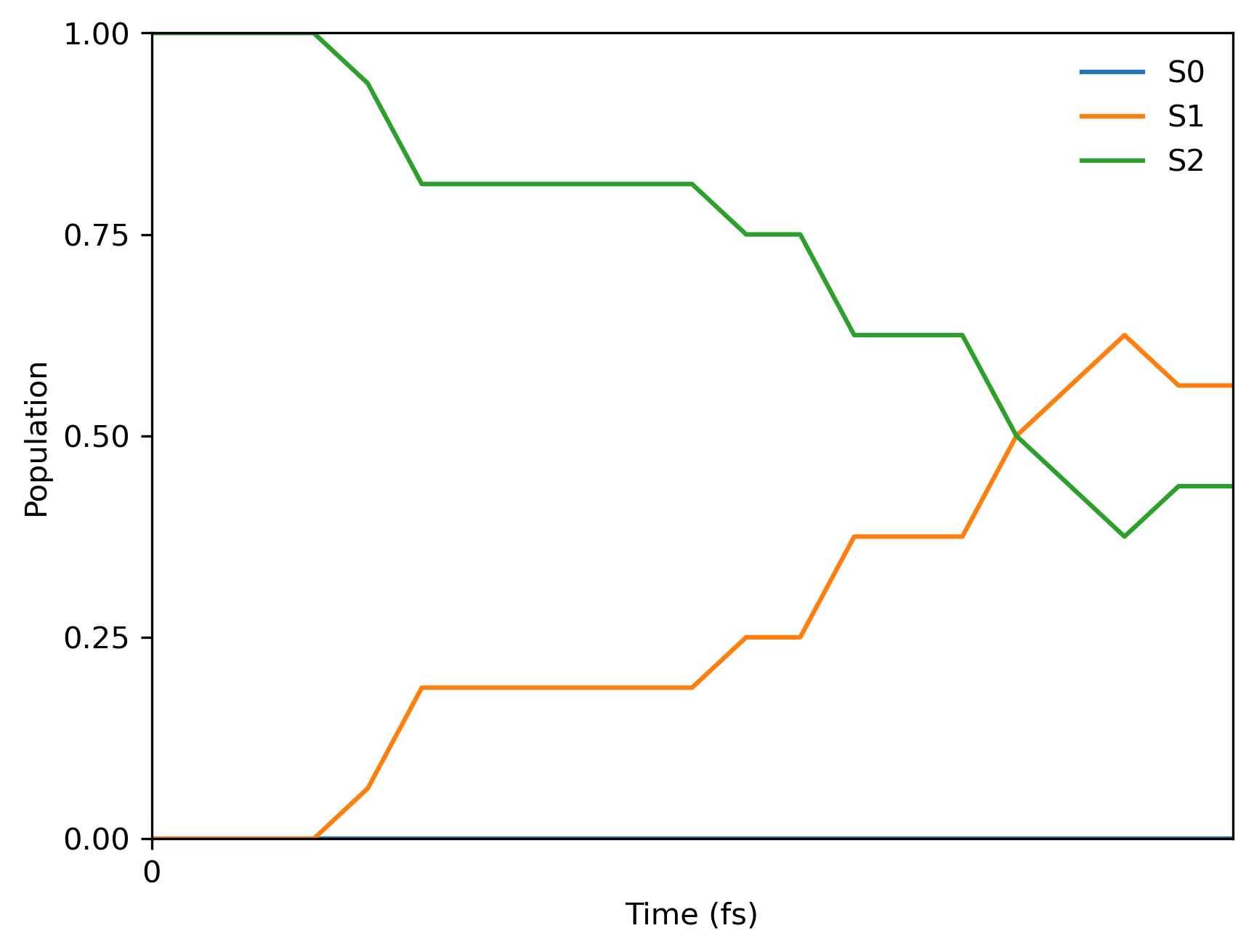
您还将获得带有群体信息的文本文件 pop.txt
,其内容应如下:
0.000 0.0 0.0 1.0
0.250 0.0 0.0 1.0
0.500 0.0 0.0 1.0
0.750 0.0 0.0 1.0
...
Analyzing results
data_analysis.ipynb
.